Details
Visual Question Answering Systems
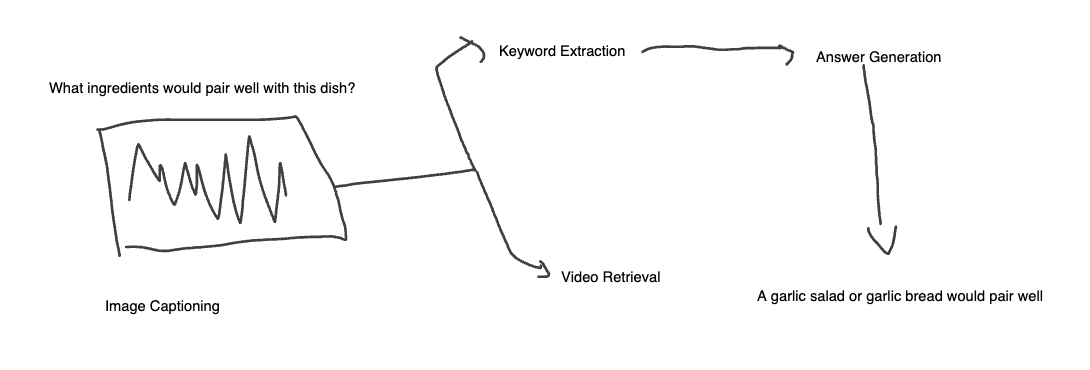
Year: 2025
Term: Winter
Student Name: Mir Hassan
Supervisor: Majid Komeili
Abstract: This research project presents a new Visual Question Answering (VQA) model that integrates image captioning, keyword extraction, web video search, and transcript-based reasoning to respond to users' questions about images. Unlike conventional VQA models constrained by pre-fixed datasets, this method imitates human behavior in actively querying YouTube for the content of interest, using BLIP for image captioning, YAKE for keyword extraction, Selenium for video scraping, GPT-4o-mini for transcript ranking, and LLaVa for answer generation. The system generates two types of responses. One uses just the image and question, and one uses external video context. While the context model was intended to increase answer quality, tests revealed that irrelevant video content and unfiltered transcripts worked to reduce accuracy compared to the base model (62.27% vs. 67.59%). These findings highlight the pitfalls of integrating real-time web data and multimodal artificial intelligence, laying the groundwork for additional research in retrieval-augmented reasoning and adaptive visual question-answering systems.