Details
Using Hand Tracking to Improve Surgical Phase Recognition
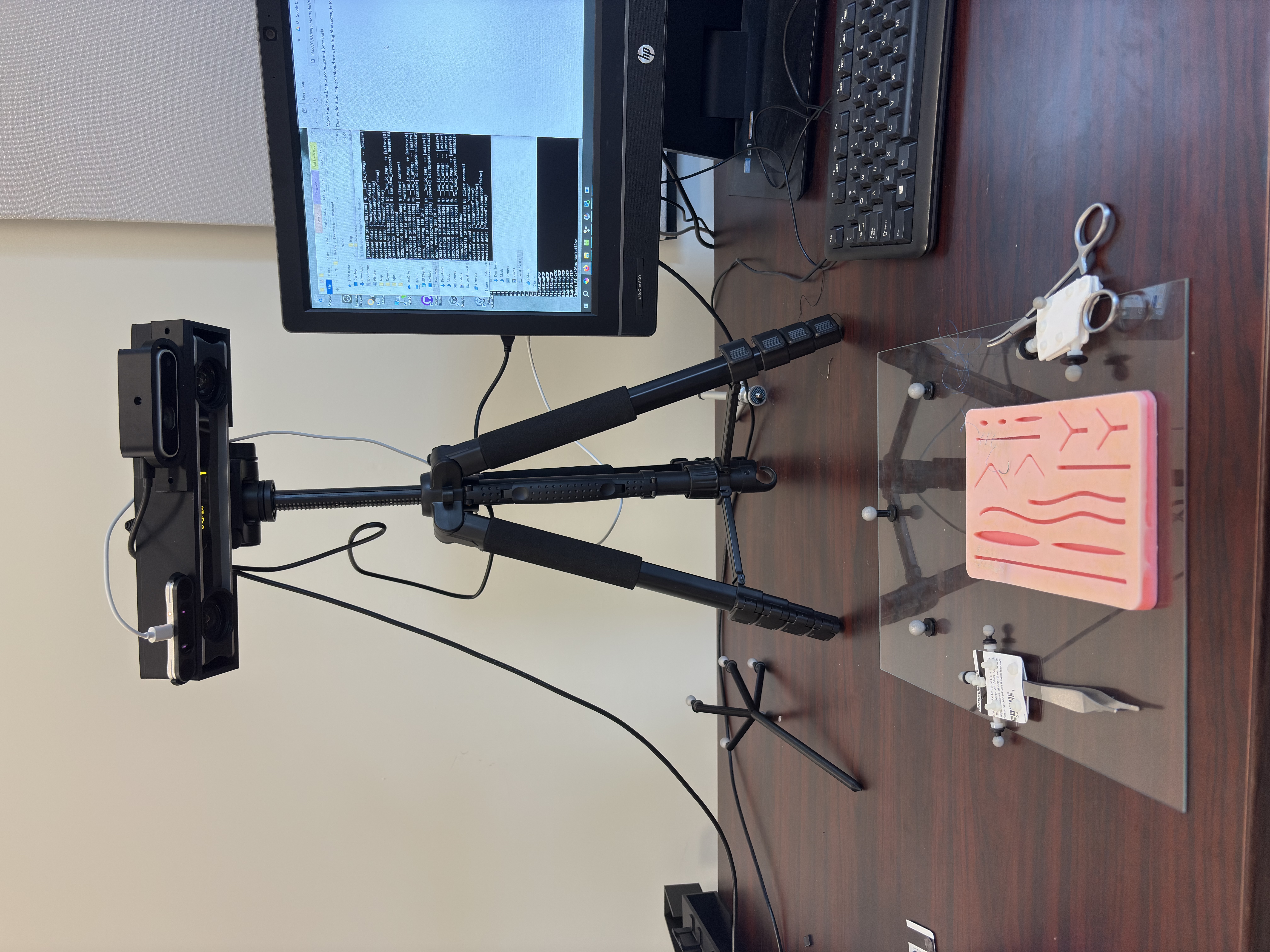
Year: 2025
Term: Winter
Student Name: Raymond Weiming Chan
Supervisor: Matthew Holden
Abstract: Surgical phase recognition provides avenues to improve real-time decision support, post-operative analysis, and surgical training. This project investigates the integration of hand-tracking data on top of instrument-tracking data. Data on simple running sutures were trained and evaluated using a Multi-Stage Temporal Convolutional Network (MS-TCN). A custom calibration pipeline aligned both modalities spatially and temporally. Results revealed instrument-tracking outperformed hand-tracking across all metrics. Combining modalities marginally improved edit score but degraded accuracy and F1 scores. While hand-tracking alone proved inadequate, its complementary role warrants further exploration with robust trackers and larger datasets. Future work should incorporate cross-validation, diverse participant data, and hyperparameter optimization to enhance generalizability. These findings show instrument-tracking’s effectiveness in phase recognition but also show the potential value hand-tracking is able to bring in.