Details
Evaluating the impact of sensor calibration on surgeon skill assessment using ultrasound needle data
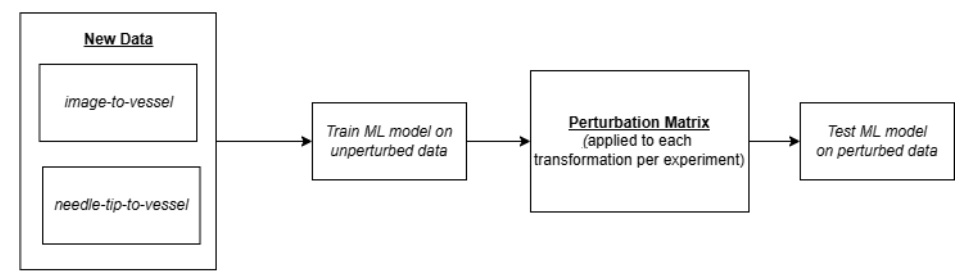
Year: 2025
Term: Winter
Student Name: Jason Au
Supervisor: Matthew Holden
Abstract: This study examines whether recalibrating sensor-based tracking systems before every surgical training session is necessary. Current systems face clinical challenges due to their non-sterilizable nature. Using a dataset of ultrasound-guided needle insertions from novices and experts, researchers introduced synthetic calibration errors to simulate real-world drift and tested how these perturbations affect the performance of deep learning models. Temporal Convolutional Networks (TCNs) were trained to predict expert skill ratings. Surprisingly, the model often maintained or even improved performance under calibration errors, particularly in out-of-plane tasks. This suggests TCNs rely more on motion patterns than precise spatial data, acting similarly to regularization. The model achieved an average mean absolute error (MAE) of 1.95—on par with human experts. These results suggest strict recalibration may not be necessary, highlighting the potential for more resilient and deployable ML-based assessment systems in clinical environments. Future work should define tolerable error thresholds and test in real-world settings.