Details
Refining State-Based Augmentation in Generative Flow Networks (GFlowNets) for Improved Learning Efficiency
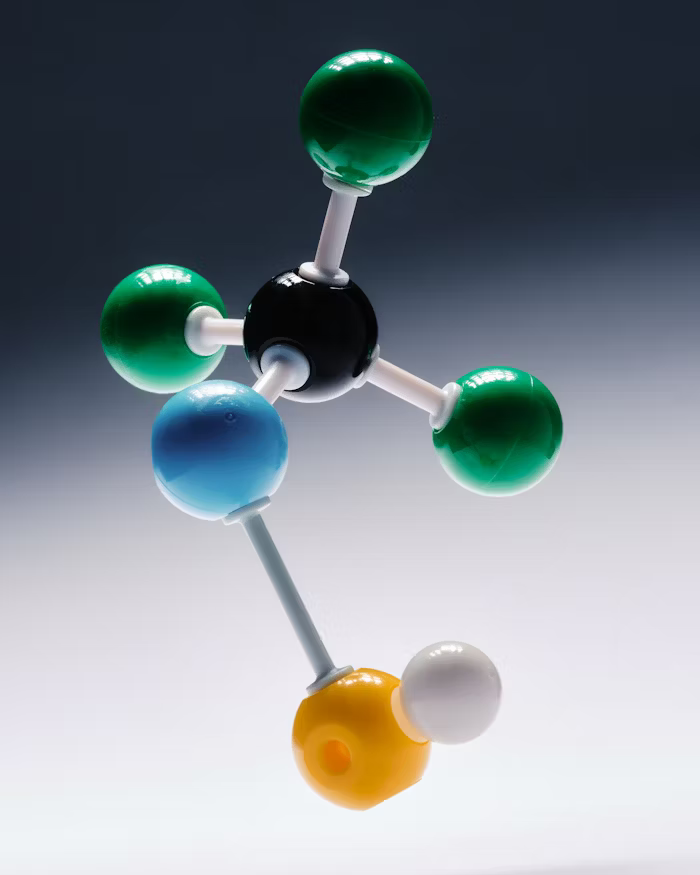
Year: 2025
Term: Winter
Student Name: Paul Hoang
Supervisor: Junfeng Wen
Abstract: This research investigates and refines state-based augmentation within Generative Flow Networks (GFlowNets) to improve learning efficiency in sparse reward environments. Through systematic experimentation with molecular generation tasks, we demonstrate that contrary to theoretical expectations, the original GFlowNet's deterministic sampling outperforms GAFlowNet's stochastic approach in discovering diverse high-reward molecular structures. Our analysis reveals that sparse reward thresholding dramatically reduces performance across all metrics, with continuous reward transformation proving more effective. Additionally, we identify stability issues with Random Network Distillation for generating intrinsic rewards and demonstrate that alternative loss functions derived from matching loss with exponential transformation significantly underperform despite theoretical promise. These findings advance our understanding of state-based augmentation limitations and provide insights for enhancing GFlowNets in challenging environments where exploration is critical for discovering high-reward solutions.