Details
Autonomous Helicopter Landing Point Range Estimation Through Flight Trajectory and Landing Site Lidar Point Cloud Using a Supervised Machine Learning Approach
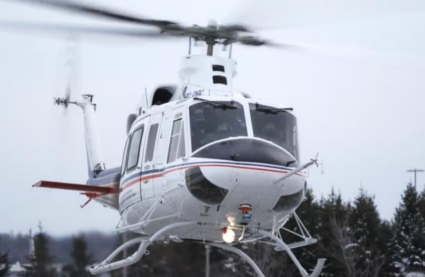
Year: 2025
Term: Winter
Student Name: Jian Zhou (Tony) Tu
Supervisor: Matthew Holden
Abstract: The Canadian Vertical Lift Autonomy Demonstration (CVLAD) team from Flight Research Laboratory (FRL) under the Nation Research Council (NRC) has been conducting research on autonomous helicopter flights using the Bell 412 Advanced Systems Research Aircraft (ASRA) as the primary platform [1]. One main feature of the project is the Landing Zone Evaluation tool (LZE), which combines data from points clouds generated by the LIDAR sensor onboard together with GPS / IMU data to identify and rank potential landing points. This allows for either pilot supervised or full autonomous landings [2]. In certain scenarios, there could be a delay in landing point identification and ranking, leading to the Bell 412 needing to make more aggressive maneuvers to adjust to the finalized landing point [2]. This paper aims to develop a solution in the form of a supervised machine learning (ML) model that is capable of predicting the distance between the the finalized landing point and the location of the helicopter when the finalized landing point is selected, which would allow the user to adjust the planned flight in relation to the finalized landing site to minimize aggressive maneuvering of the Bell 412 Helicopter.