Details
Analyzing the Integration of Ethical Principles in AI/ML Workflows
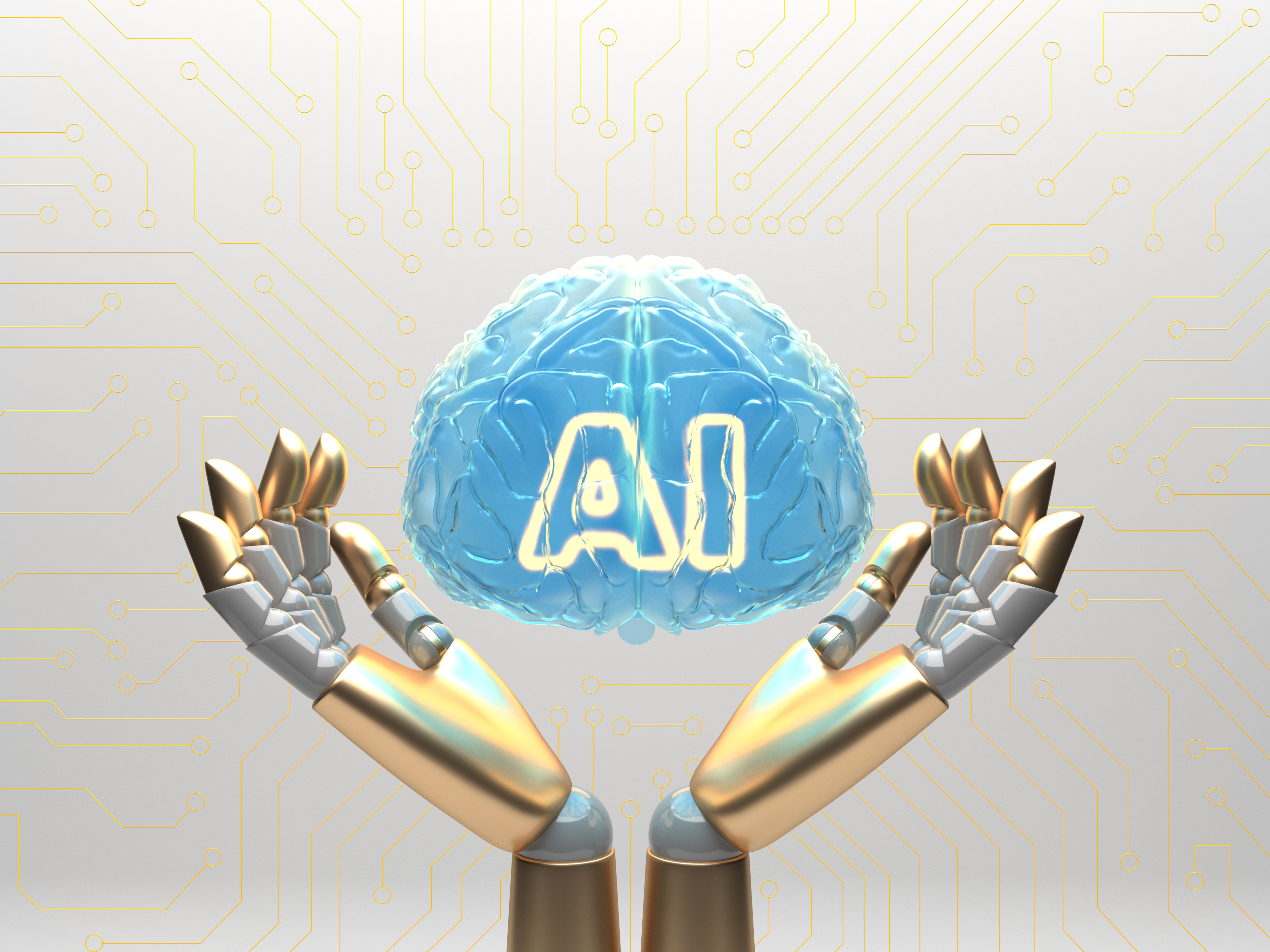
Year: 2025
Term: Winter
Student Name: Sai Male
Supervisor: Olga Baysal
Abstract: The integration of ethical principles into artificial intelligence and machine learning (AI/ML) workflows has become a critical concern, yet evidence of their practical application remains limited. This thesis investigates how ethical principles are reflected in real-world AI/ML development by analyzing 252,574 closed GitHub issues across 28 repositories. Using a curated set of ethics-related keywords, the study estimates the rate of ethical integration at 11.47%. To evaluate the effectiveness of automated analysis, two large language models (LLMs) were tested on a manually labeled subset of 400 issues categorized by the EU’s seven ethical principles. Among all tested configurations, Llama 3.3 using a structured prompt at a temperature of 0.2 achieved the best classification performance, with an F1 score of 0.7453. However, class imbalance led to frequent overprediction of the most dominant principle, Technical Robustness and Safety, which accounted for over 60% of all labeled issues. In addition to the computational evaluation, a questionnaire was developed to explore developers’ awareness of and challenges with applying ethical principles. Although the questionnaire was not deployed, it lays the groundwork for future empirical research. The findings highlight an ongoing gap between ethical intentions and actual practice in AI/ML workflows. While LLMs show potential for supporting ethics-related analysis, they must be complemented by human insight to ensure a more balanced and comprehensive approach to ethical AI/ML development.