Details
Synthetic Data Generation Techniques and Their Application in Machine Learning for Effective Fraud Detection
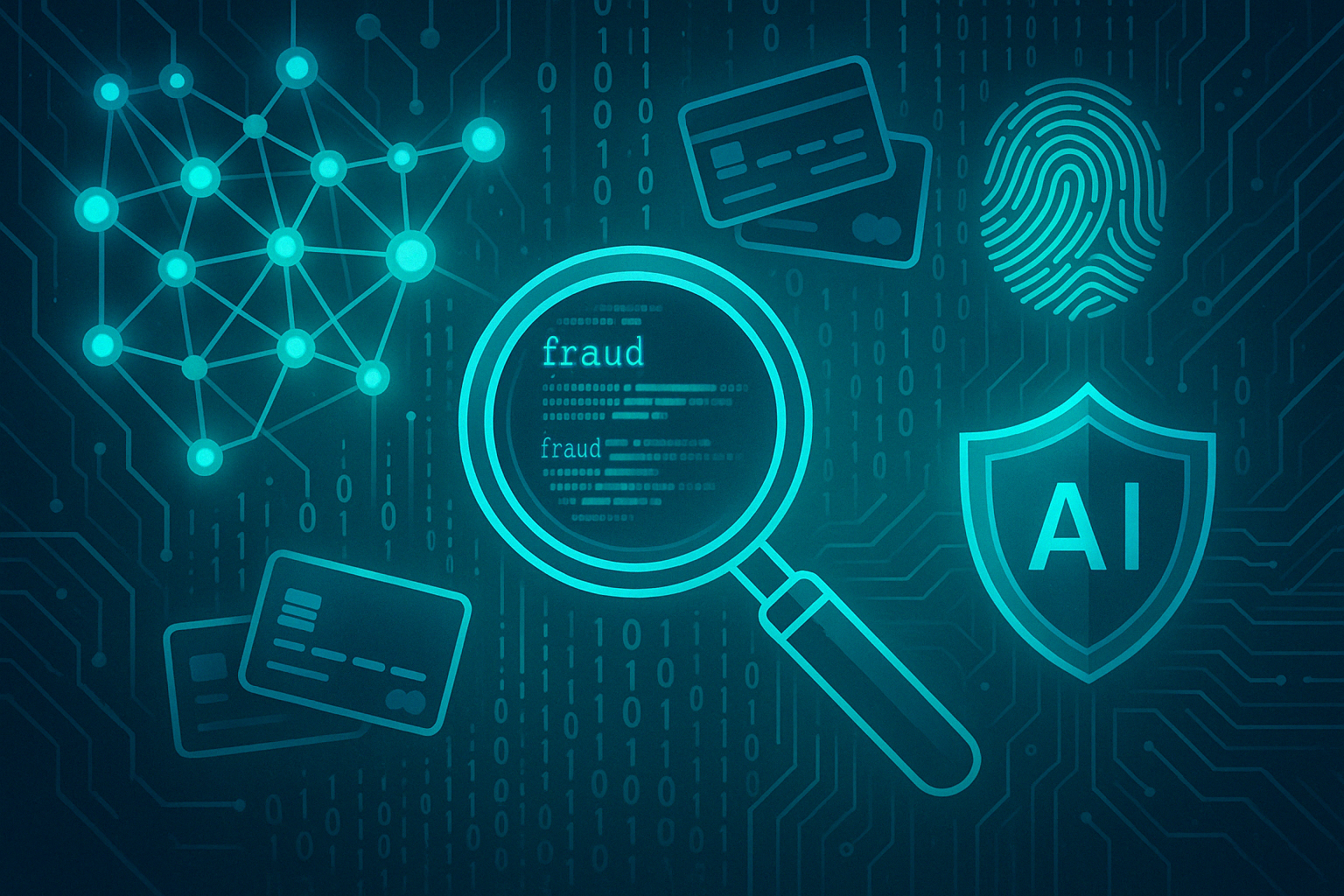
Year: 2025
Term: Winter
Student Name: Rosa Li
Supervisor: Anil Somayaji
Abstract: The growing prevalence of fraud presents a significant challenge. It is costing billions of dollars a year for financial institutions, e-commerce providers and vendors. This calls for robust and adaptable detection systems. The problem of fraud detection is one of anomaly detection as the number of fraudulent cases, although can be large, is small proportional to the total number of cases. This thesis explores the empirical understanding of algorithmic behavior in fraud detection through constructing synthetic datasets. While prior research has extensively analyzed machine learning algorithms for fraud detection, synthetic data generation and fraud patterns, there is a notable gap in the area of understanding algorithmic behaviour using synthetic data generation tailored to fraud detection. To address this gap, this study explores constructing synthetic datasets to better understand the boundaries of machine learning models. By evaluating the performance of the selected models on these datasets, this research aims to provide insights into their efficacy and adaptability in real-world fraud detection scenarios. The findings contribute to the development of more sophisticated fraud detection systems, enhancing both the accuracy and robustness of predictive models.